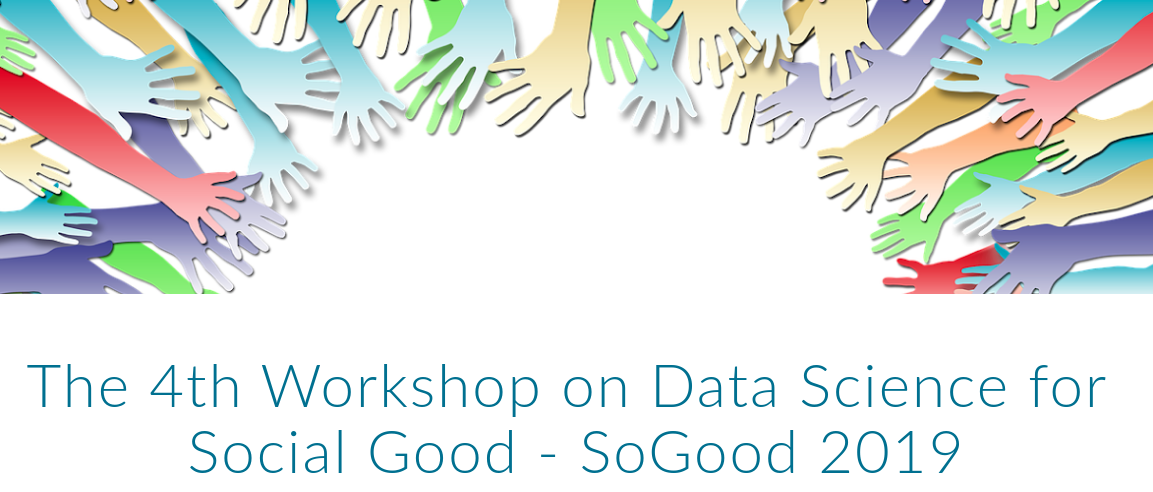
We are very pleased to announce that our group got 2 papers accepted at the 4th Workshop on Data Science for Social Good.
SoGood is a peer-reviewed workshop that focuses on how Data Science can and does contribute to social good in its widest sense. The workshop is held from 2016 yearly together with ECML PKDD Conference and this year is on 20th September, Wurzburg, Germany.
Here is the pre-print of the accepted papers with their abstract:
- Linking Physicians to Medical Research Results via Knowledge Graph Embeddings and Twitter by Afshin Sadeghi and Jens Lehmann.
Abstract: Informing professionals about the latest research results in their field is a particularly important task in the field of health care, since any development in this field directly improves the health status of the patients. Meanwhile, social media is an infrastructure that allows public instant sharing of information, thus it has recently become popular in medical applications. In this study, we apply Multi Distance Knowledge Graph Embeddings (MDE) to link physicians and surgeons to the latest medical breakthroughs that are shared as the research results on Twitter. Our study shows that using this method physicians can be informed about the new findings in their field given that they have an account dedicated to their profession. - Improving Access to Science for Social Good by Mehdi Ali, Sahar Vahdati, Shruti Singh, Sourish Dasgupta, and Jens Lehmann.
Abstract: One of the major goals of science is to make the world socially a good place to live. The old paradigm of scholarly communication through publishing has generated enormous amount of heterogeneous data and metadata. However, most scientific results are not easy to discover, in particular those results which benefit social good and are also targeted at non-scientific people. In this paper, we showcase a knowledge graph embedding (KGE) based recommendation system to be used by students involved in activities aiming at social good. The recommendation system has been trained on a scholarly knowledge graph, which we constructed. The obtained results highlight that the KGEs successfully encoded the structure of the KG, and therefore, our system could provide valuable recommendations.
Acknowledgement
This study is partially supported by project MLwin (Maschinelles Lernen mit Wissensgraphen, grant no. 01IS18050F), Cleopatra (grant no. 812997), EPSRC grant EP/M025268/1, the WWTF grant VRG18-013, LAMBDA (GA no. 809965). The authors gratefully acknowledge financial support from the Federal Ministry of Education and Research of Germany (BMBF) which is funding MLwin and European Union Marie Curie ITN that funds Cleopatra, as well as Fraunhofer IAIS.