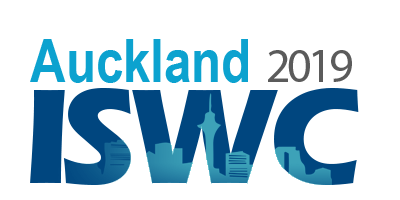
We are very pleased to announce that our group got 7 demo/poster papers accepted for presentation at ISWC 2019: the 18th International Semantic Web Conference, which will be held on October 26 – 30 2019 in Auckland, New Zealand.
The International Semantic Web Conference (ISWC) is the premier international forum where Semantic Web / Linked Data researchers, practitioners, and industry specialists come together to discuss, advance, and shape the future of semantic technologies on the web, within enterprises and in the context of the public institution.
Here is the list of the accepted papers with their abstract:
- “Querying large-scale RDF datasets using the SANSA framework” by Claus Stadler, Gezim Sejdiu, Damien Graux, and Jens Lehmann.
Abstract: In this paper, we present Sparklify: a scalable software component for efficient evaluation of SPARQL queries over distributed RDF datasets. In particular, we demonstrate a W3C SPARQL endpoint powered by our SANSA framework’s RDF partitioning system and Apache SPARK for querying the DBpedia knowledge base. This work is motivated by the lack of Big Data SPARQL systems that are capable of exposing large-scale heterogeneous RDF datasets via a Web SPARQL endpoint.
- “How to feed the Squerall with RDF and other data nuts?” by Mohamed Nadjib Mami, Damien Graux, Simon Scerri, Hajira Jabeen, Sören Auer, and Jens Lehmann.
Abstract: Advances in Data Management methods have resulted in a wide array of storage solutions having varying query capabilities and supporting different data formats. Traditionally, heterogeneous data was transformed off-line into a unique format and migrated to a unique data management system, before being uniformly queried. However, with the increasing amount of heterogeneous data sources, many of which are dynamic, modern applications prefer accessing directly the original fresh data. Addressing this requirement, we designed and developed Squerall, a software framework that enables the querying of original large and heterogeneous data on-the-fly without prior data transformation. Squerall is built from the ground up with extensibility in consideration, e.g., supporting more data sources. Here, we explain Squerall’s extensibility aspect and demonstrate step-by-step how to add support for RDF data, a new extension to the previously supported range of data sources.
- “Towards Semantically Structuring GitHub” by Dennis Oliver Kubitza, Matthias Böckmann, and Damien Graux.
Abstract: With the recent increase of open-source projects, tools have emerged to enable developers collaborating. Among these, git has received lots of attention and various on-line platforms have been created around this tool, hosting millions of projects. Recently, some of these platforms opened APIs to allow users questioning their public databases of open-source projects. Despite the common protocol core, there are for now no common structures someone could use to link those sources of information. To tackle this, we propose here the first ontology dedicated to the git protocol and also describe GitHub’s features within it to show how it is extendable to encompass more git-based data sources.
- “Microbenchmarks for Question AnsweringSystems Using QaldGen” by Qaiser Mehmood, Abhishek Nadgeri, Muhammad Saleem, Kuldeep Singh, Axel-Cyrille Ngonga Ngomo and Jens Lehmann.
Abstract: [Microbenchmarks are used to test the individual components of the given systems. Thus, such benchmarks can provide a more detailed analysis pertaining to the different components of the systems. We present a demo of the QaldGen, a framework for generating question samples for micro-benchmarking of Question Answering (QA) systems over Knowledge Graphs (KGs). QaldGen is able to select customized question samples from existing QA datasets. The sampling of questions is carried out by using different clustering techniques. It is flexible enough to select benchmarks of varying sizes and complexities according to user-defined criteria on the most important features to be considered for QA benchmarking. We evaluate the usability of the interface by using the standard system usability scale questionnaire. Our overall usability score of 77.25 (ranked B+) suggests that the online interface is recommendable easy to use, and well-integrated.
- “ FALCON: An Entity and Relation Linking framework over DBpedia” by Ahmad Sakor, Kuldeep Singh, Maria Esther Vidal.
Abstract: [We tackle the problem of entity and relation linking and present FALCON, a rule-based tool able to accurately map entities and relations in short texts to resources in a knowledge graph. FALCON resorts to fundamental principles of the English morphology (e.g., compounding and headword identification) and performs joint entity and relation linking against a short text. We demonstrate the benefits of the rule-based approach implemented in FALCON on short texts composed of various types of entities. The attendees will observe the behavior of FALCON on the observed limitations of Entity Linking (EL) and Relation Linking (RL) tools. The demo is available at https://labs.tib.eu/falcon/.
- “Demonstration of a Customizable Representation Model for Graph-Based Visualizations of Ontologies – GizMO” by Vitalis Wiens, Mikhail Galkin, Steffen Lohmann, and Sören Auer
Abstract: Visualizations can facilitate the development, exploration, communication, and sense-making of ontologies. Suitable visualizations, however, are highly dependent on individual use cases and targeted user groups. In this demo, we present a methodology that enables customizable definitions for ontology visualizations. We showcase its applicability by introducing GizMO, a representation model for graph-based visualizations in the form of node-link diagrams. Additionally, we present two applications that operate on the GizMO representation model and enable individual customizations for ontology visualizations.
- “Predict Missing Links Using PyKEEN“ by Mehdi Ali, Charles Tapley Hoyt, Daniel Domingo-Fernandez, and Jens Lehmann.
Abstract:PyKEEN is a framework, which integrates several approaches to compute knowledge graph embeddings (KGEs). We demonstrate the usage of PyKEEN in a biomedical use case, i.e. we trained and evaluated several KGE models on a biological knowledge graph containing genes’ annotations to pathways and pathway hierarchies from well-known databases. We used the best performing model to predict new links and present an evaluation in collaboration with a domain expert.
Acknowledgement
This work has received funding from the EU Horizon 2020 projects BigDataOcean (GA no. 732310), Boost4.0 (GA no. 780732), SLIPO (GA no. 731581) and QROWD (GA no. 723088).
Looking forward to seeing you at The ISWC 2019.